Transform Your Data Strategy with Our Image Annotation Tool for Machine Learning
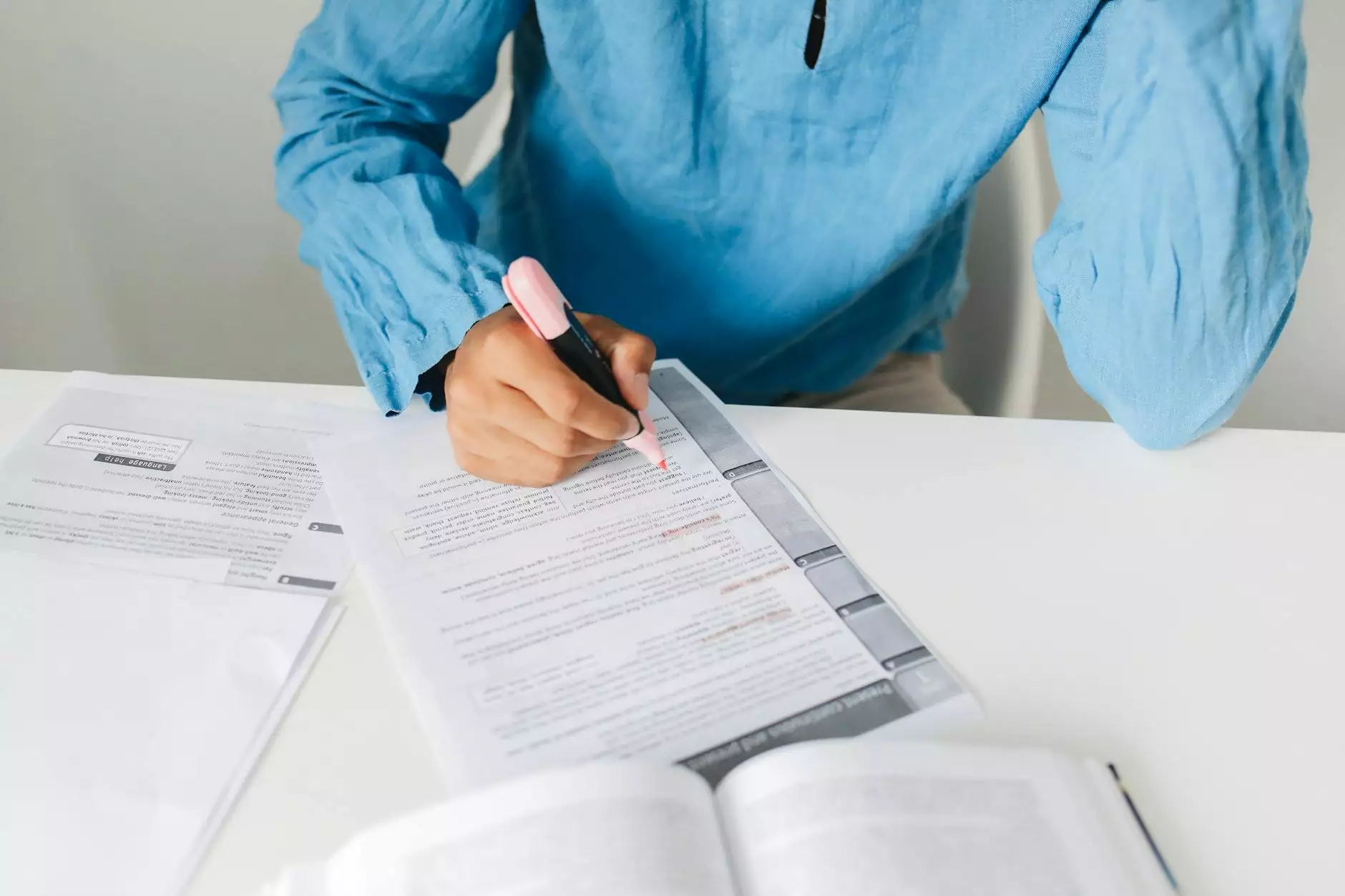
In today's rapidly advancing technological landscape, businesses are continually seeking innovative solutions to optimize their operations and improve their data strategies. One such solution involves the use of an image annotation tool machine learning designed to streamline the data annotation process. KeyLabs AI specializes in offering cutting-edge data annotation tools and platforms that empower companies to harness the full potential of their image data.
Understanding Image Annotation and Its Importance
Image annotation refers to the process of labeling images to make them understandable for machine learning algorithms. It involves tagging objects, features, and relevant information within images, which is essential for training AI models. Here are a few key reasons why image annotation is vital in machine learning:
- Improves Model Accuracy: Accurate annotations help algorithms understand the nuances of various objects within an image, leading to better predictive accuracy.
- Enables Supervised Learning: For machine learning to yield reliable results, models require annotated data to learn from.
- Facilitates Diverse Applications: Image annotation supports a wide range of applications such as facial recognition, autonomous driving, and medical image analysis.
The Evolution of Image Annotation Tools
With the emergence of deep learning and artificial intelligence, traditional image annotation methods have undergone significant transformation. The evolution of image annotation tools has led to increased efficiency and improved capabilities. Historical methods relied heavily on manual labor, which was time-consuming and prone to errors. However, advancements in technology have ushered in automated and semi-automated systems. This evolution is crucial for industries aiming to leverage large datasets for machine learning applications.
Types of Image Annotation
Image annotation can be categorized into several types, each serving distinct purposes:
- Bounding Box Annotation: In this technique, rectangular boxes are drawn around objects to help the model learn to identify them.
- Polygon Annotation: This method outlines the shape of an object more accurately than bounding boxes, making it useful for complex shapes.
- Semantic Segmentation: Each pixel in the image is classified into categories, providing detailed understanding of the image content.
- Image Tagging: Simple tags are added to images based on their content, aiding in organization and searchability.
Key Features of an Effective Image Annotation Tool for Machine Learning
A robust image annotation tool machine learning should possess several essential features to enhance the data preparation process:
1. User-Friendly Interface
A clear and intuitive interface allows users, regardless of their technical expertise, to navigate the tool easily and efficiently.
2. Variety of Annotation Options
The tool should offer multiple annotation types, enabling users to choose the most appropriate method based on their specific needs.
3. Automatic Annotation Capabilities
Integrating AI-driven automatic annotations can significantly reduce manual effort and time, increasing annotation productivity.
4. Collaboration Features
Collaborative tools allow teams to annotate images in real-time, ensuring streamlined communication and consistency in the annotation process.
5. Quality Control Mechanisms
Implementing quality control checks ensures the accuracy and reliability of annotations, which is vital for effective machine learning outcomes.
The Role of KeyLabs AI in Image Annotation
At KeyLabs AI, we provide a state-of-the-art data annotation platform that simplifies and enhances the image annotation process for machine learning. Our comprehensive toolset offers a range of features tailored to meet the diverse needs of businesses across various industries.
Enhancing Productivity with KeyLabs AI
Our image annotation tool is designed for efficiency. By utilizing intelligent automation features and straightforward workflows, companies can boost their productivity significantly. Some of the standout aspects of our platform include:
- Scalable Solutions: Whether you have a small project or large-scale enterprise needs, our platform scales with you.
- Real-time Collaboration: Annotators can work together from different locations, enhancing team productivity and output quality.
- Integration with Popular Machine Learning Frameworks: Seamlessly connect with ML frameworks like TensorFlow and PyTorch to streamline your data pipeline.
Use Cases of Image Annotation in Machine Learning
The application of image annotation is vast and varied. Here are some prominent use cases:
1. Autonomous Vehicles
In the realm of self-driving cars, image annotation plays a pivotal role. Annotated images help train algorithms to recognize road signs, pedestrians, and other vehicles, facilitating safe navigation.
2. Healthcare and Medical Imaging
In healthcare, image annotation can assist in diagnosing conditions through medical imaging. For instance, annotated MRI images can help radiologists and AI models identify tumors with greater accuracy.
3. Retail and E-commerce
Businesses can leverage image annotation for product recognition in retail applications. This enables features like visual search, wherein customers can upload images to find similar products.
4. Agriculture Monitoring
In agriculture, annotated drone imagery can help farmers monitor crop health, detect pests, and assess soil conditions, ultimately leading to better crop management practices.
Challenges in Image Annotation
Despite the advantages, the image annotation process poses several challenges:
- Consistency and Accuracy: Maintaining consistency in annotations across large datasets can be difficult, often leading to discrepancies that affect model performance.
- Time Consumption: Even with automation, extensive annotation tasks can take significant time, delaying project timelines.
- Skill Requirements: Some annotation tasks require specific domain knowledge to ensure high-quality annotations.
Best Practices for Effective Image Annotation
To overcome these challenges and make the most of your annotation efforts, consider the following best practices:
1. Define Clear Guidelines
Establishing comprehensive annotation guidelines ensures that all team members interpret and annotate images consistently, leading to a more reliable dataset.
2. Leverage Automation Wisely
Utilize automatic annotation tools to reduce manual workloads, but always include a human review process to ensure quality.
3. Conduct Regular Quality Checks
Instituting quality control checkpoints throughout the annotation process helps catch errors early, minimizing the need for extensive rework.
Conclusion: Embrace the Future of Data with KeyLabs AI
Embracing a sophisticated image annotation tool for machine learning can significantly elevate your data strategy. With KeyLabs AI's innovative solutions, businesses can efficiently annotate vast amounts of image data and generate highly accurate models that provide competitive advantages. Dive into the future of automated data annotation, improve your machine learning outcomes, and propel your business to new heights with our dedicated platform.
For more information on how to get started with our data annotation platform and enhance your machine learning projects, visit KeyLabs AI today!